数据标准化是处理不同规模和量纲数据的方式,使其缩放到相同的数据区间和范围,以减少规模、特征、分布差异等对模型的影响。比如:员工数量的值是 50 - 2000 人,销售额的值是 1000000 - 5000000 万。单位尺度不同,使用梯度下降算法,就需要很多次迭代。
Max-Min 标准化方法是对原始数据进行线性变换,假设原转换的数据为 x,新数据为 x′,那么 x’=(x-min)/(max-min),其中 min 和 max 为 x 所在列的最小值和最大值。得到的数据会完全落入 [0, 1] 区间内。
Python 中的 sklearn 库使用起来比较方便,接下来还是演示下。
1 2 3 4 5 6
| import seaborn as sns from pandas import Series,DataFrame import pandas as pd import matplotlib.pyplot as plt %matplotlib inline
|
1 2 3 4 5 6 7
| tips = sns.load_dataset('tips')
tips.head()
|
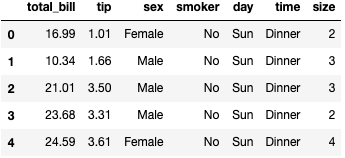
1 2
| tips = tips.drop(['sex','smoker','day','time'],axis=1) tips.head()
|
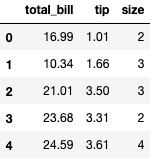
1 2 3 4
| from sklearn.preprocessing import MinMaxScaler scaler = MinMaxScaler() result = scaler.fit_transform(tips) result[:5]
|
1 2 3 4 5
| array([[0.29157939, 0.00111111, 0.2 ], [0.1522832 , 0.07333333, 0.4 ], [0.3757855 , 0.27777778, 0.4 ], [0.43171345, 0.25666667, 0.2 ], [0.45077503, 0.29 , 0.6 ]])
|
通过这个演示也可以看到,Max-Min标准化只能用在数值型特征上,不适用在分类变量。