数据选取的 4 种方式:
- 使用 loc() 和 iloc() 选取单独几行
- 使用 isin() 查找和选取对应数据
- 使用 unique() 选出唯一值
- 使用 df.nlargest() 和 df.nsmallest()
使用loc和iloc选取单独几行
使用loc 按行索引标签选取数据
1 2 3 4 5 6 7
| import pandas as pd import numpy as np df = pd.DataFrame({'A': pd.date_range('2019/01/01',periods=6), 'B': ['a','b','c','d','e', 'f'], 'C': np.arange(10, 16)}) df = df.set_index('A') df
|
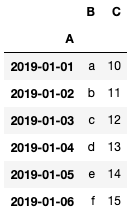
1 2 3 4 5
|
df.loc['2019-01-01':'2019-01-03']
|
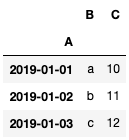
1 2
| df.loc['2019-01-01':'2019-01-03','B' ]
|
1 2 3 4 5
| A 2019-01-01 a 2019-01-02 b 2019-01-03 c Name: B, dtype: object
|
1 2 3 4 5
|
df.loc[df['B']=='c']
|
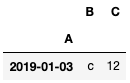
使用iloc按索引位置选取数据
1 2 3
| B a C 10 Name: 2019-01-01 00:00:00, dtype: object
|
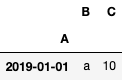
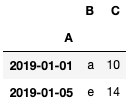
使用isin()查找和选取对应数据
1 2 3
| df = pd.DataFrame({'A': pd.date_range('2019/01/01',periods=5), 'B': ['a','b','c','d','e']}) df
|
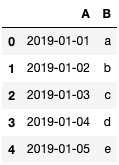
1 2 3
| data = ['2019-01-01', '2019-01-03'] df = df.loc[df['A'].isin(data), ['A','B']] df
|
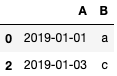
使用unique()选出唯一值
1 2 3 4 5 6
| import numpy as np A = [1, 2, 2, 5,3, 4, 3]
a = np.unique(A) a
|
1 2 3
| a, s= np.unique(A, return_index=True) s
|
1 2 3
| a, s, p = np.unique(A, return_index=True, return_inverse=True) p
|
1
| array([0, 1, 1, 4, 2, 3, 2])
|
使用 df.nlargest() 和 df.nsmallest()
在之前的实现方式,df.head() 用来查看前多少行数据,然后需要找到最大的话,往往分两步,把 df 进行排序,然后选择前多少行数据。而这两个函数分别是取df最大的前几个,和最小的前几个,比较实用。
参数解释
tips.nlargest(n, columns, keep=’first’)
n
:前xx个,int值
columns
:列名
keep='first'
:keep=’first’或者’last’。当出现重复值时,keep=’first’,会选取在原始DataFrame里排在前面的数据,keep=’last’则去排后面的数据。
还是拿小费数据集演示下。
1 2 3 4 5 6 7 8 9 10 11 12 13 14
| import seaborn as sns from pandas import Series,DataFrame import pandas as pd import matplotlib.pyplot as plt %matplotlib inline
tips = sns.load_dataset('tips')
tips.head()
|
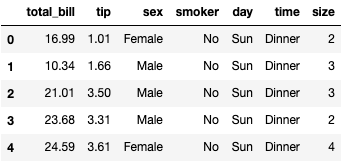
1 2
| tips.nlargest(5,'total_bill')
|
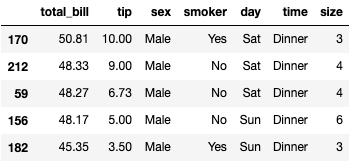
1 2
| tips.nsmallest(5,'total_bill',keep='last')
|
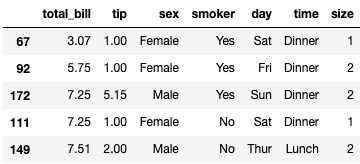